Paloma Sodhi
paloma.sodhi at gmail dot com
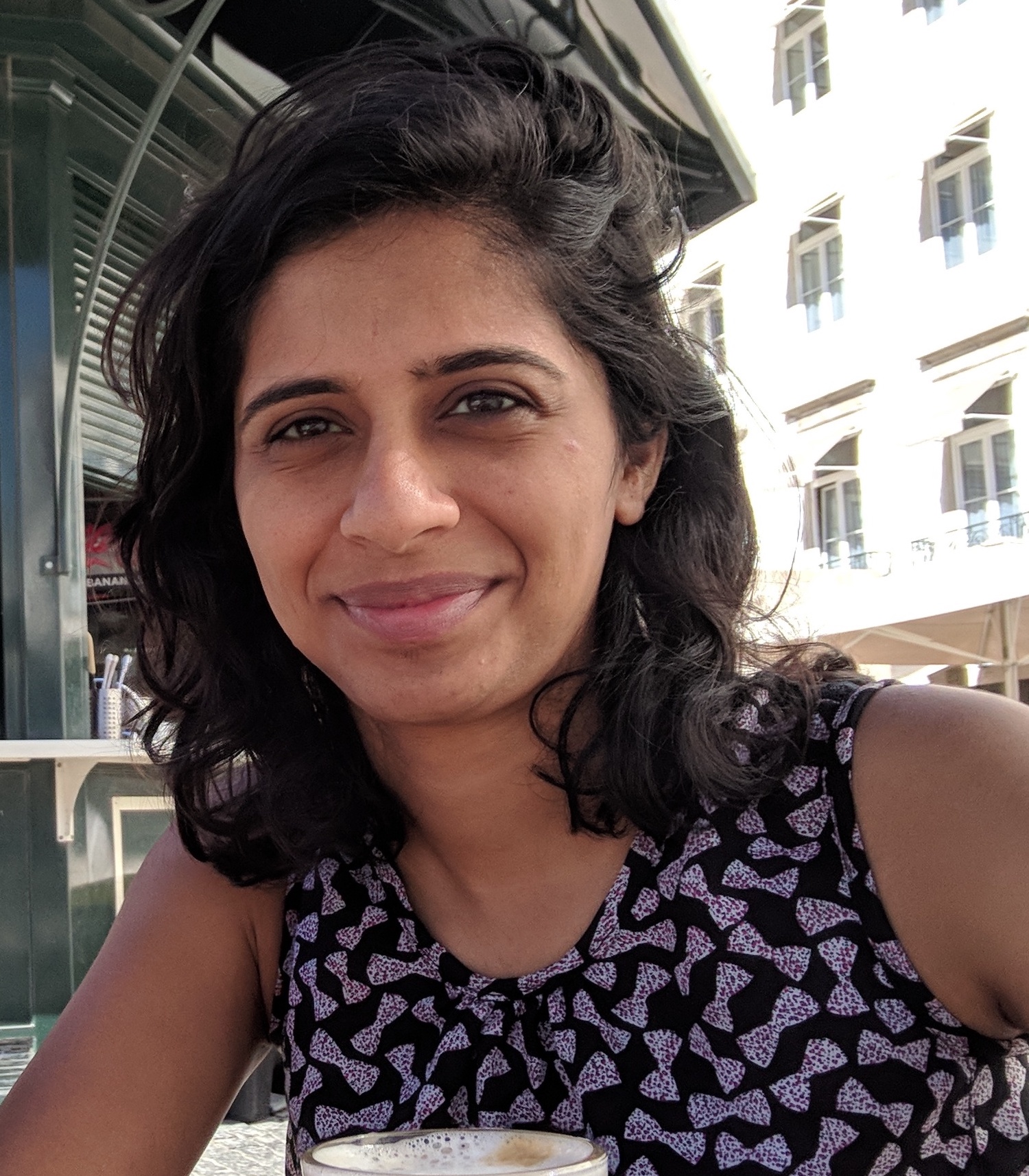
Hello! I’m a researcher at OpenAI, where I work on building next-generation LLMs to be more capable and aligned with human intents. Previously, I was a researcher at ASAPP, where I was building dialog chatbots that converse and take actions, such as interacting with external tools and user interfaces.
Overall, I am excited about technologies that free up humans from mundane tasks, helping them be more creative and better decision makers. I did my Ph.D. in Robotics at Carnegie Mellon University on combining machine learning with graph optimizers in the loop, with applications to robot manipulation.